In this post, I’ll explore the potential societal consequences of the advent of generative AI (GenAI), which includes large language models (LLMs), text to image generation models (TIG) and other generative AI models that produce high-quality content based on human instruction (prompts).
Over the last few months, as I have intensively worked on GenAI models, I have spent a lot of time grappling with questions of why generative AI is disruptive, how to best think about it, and what the societal consequences are of its general availability.
I have finally reached a point where I feel I have a good high-level mental framework to think about these questions. So today I would like to share it with you in the hope that you find it useful and that it will spark interesting conversations. More than ever, please provide feedback, as this is a complex and rapidly developing topic that we can only begin to understand together through inclusive discussions.
Disclaimer: This post represents my personal opinion on the subject, not the views of my employer (Google). Also, as you would expect, this post was written in collaboration with GenAI tools for text editing (ChatGPT4 and Bard) and image creation purposes (Midjourney)
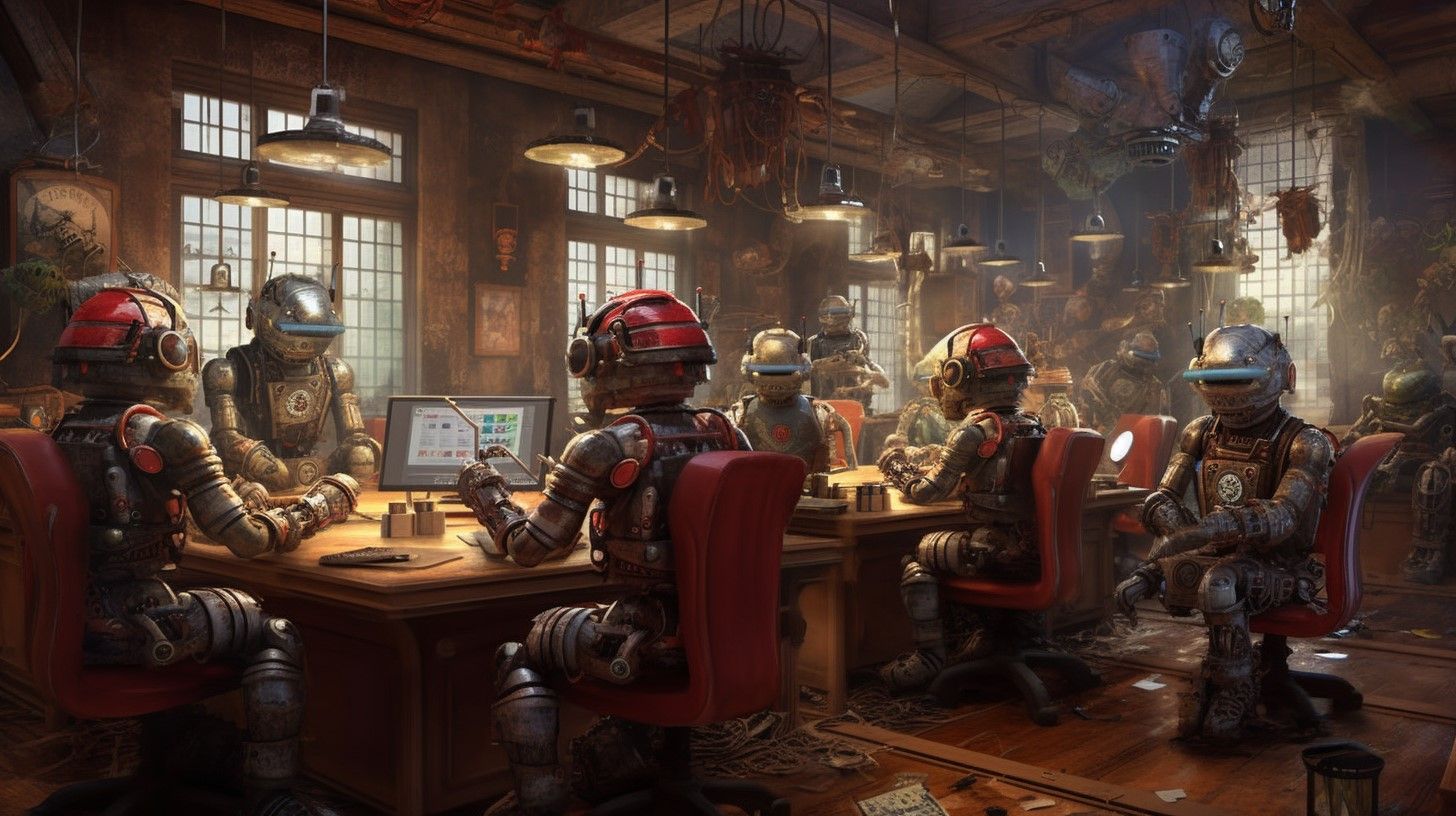
Overall, I argue that:
The best way to think about the advent of GenAI is to view it as the AI workforce entering the labor market.
Thinking about it this way makes it clear why GenAI is going to be a true societal revolution: We now have an army of cheap, capable AI labor entering the market all at once. This new workforce will disrupt the economy, businesses, and the world in the same way globalization did, but faster.
This post explores this framework by answering the following questions in turn:
- What will likely be the societal impact of the AI workforce?
- Why is using an AI workforce so appealing?
- What risks and challenges will arise along the way to this adoption?
- Balancing everything discussed in this post, what is the takeaway?
AI workforce impact
Casting AI as a new workforce leads me to rephrase the burning question that seems to be on everyone’s mind:
Long term
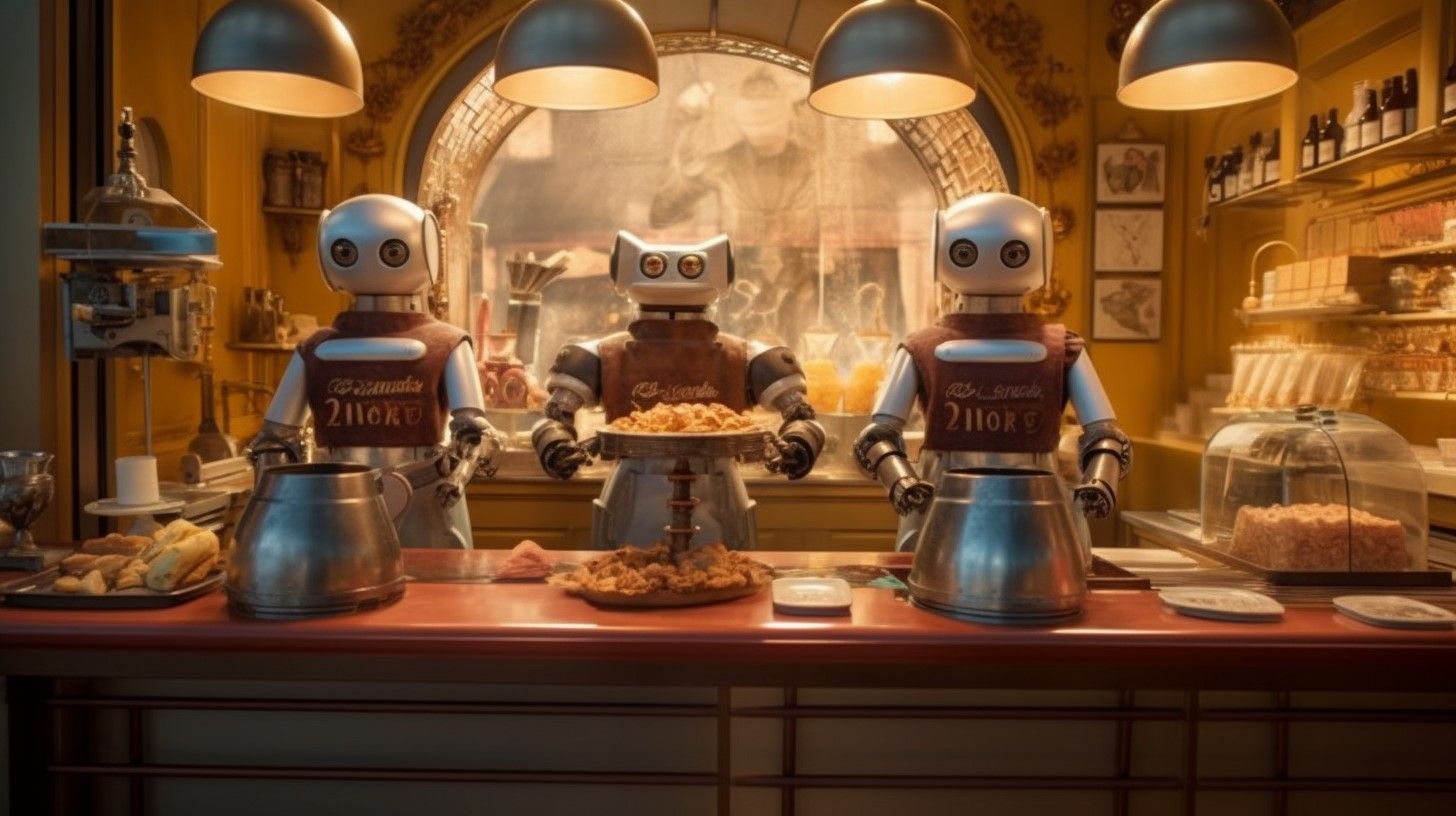
In the long term, I am optimistic that the disruption caused by AI entering the workforce will be a net benefit to humanity and increase market size rather than replacing humans. The main reason is that, between an aging human population and the increased need for diverse services due to humanity getting wealthier, we are bound to experience a shortage of human workers. IMHO, the only viable option to address this impending shortage is to have the AI workforce do the heavy lifting for us in the mid- to long-term.
The human labor shortage is closer than most people think: The world population will peak at around 9.7 billion in 2064, according to recent research.
But the effect of population shrinkage will be felt far sooner: By 2030, more than 85 million jobs might be unfilled in the U.S., including 10M health-care-related jobs because there aren’t enough skilled people to fill them. By 2050, Japan’s workforce will have shrunk from 128M to 100M due to an aging population, and by 2100, China might have more people outside the working age than in it.
This shrinking workforce, if not compensated for using AI, will reduce global production, leading to a poorer world, preventing us from supporting our aging population, and further increasing inequality, as many services commonly available today will become (even more) unaffordable to most.
If we hope to keep raising living standards across the world, the challenge will become even more insurmountable without AI workers, because we will have to drastically increase production instead of simply maintaining current production levels, which is already not a done deal. Clearly, robots will have to farm, manufacture, and transport goods, if we have any hope to end malnutrition and have all humans experience the affordance of modern technologies and benefit from great education and healthcare.
This is why I strongly believe that there is a pressing need for the AI revolution to happen if we want to escape humanity’s slow decline and the inability to innovate that comes with it — if we want to escape once again the Malthusian trap.
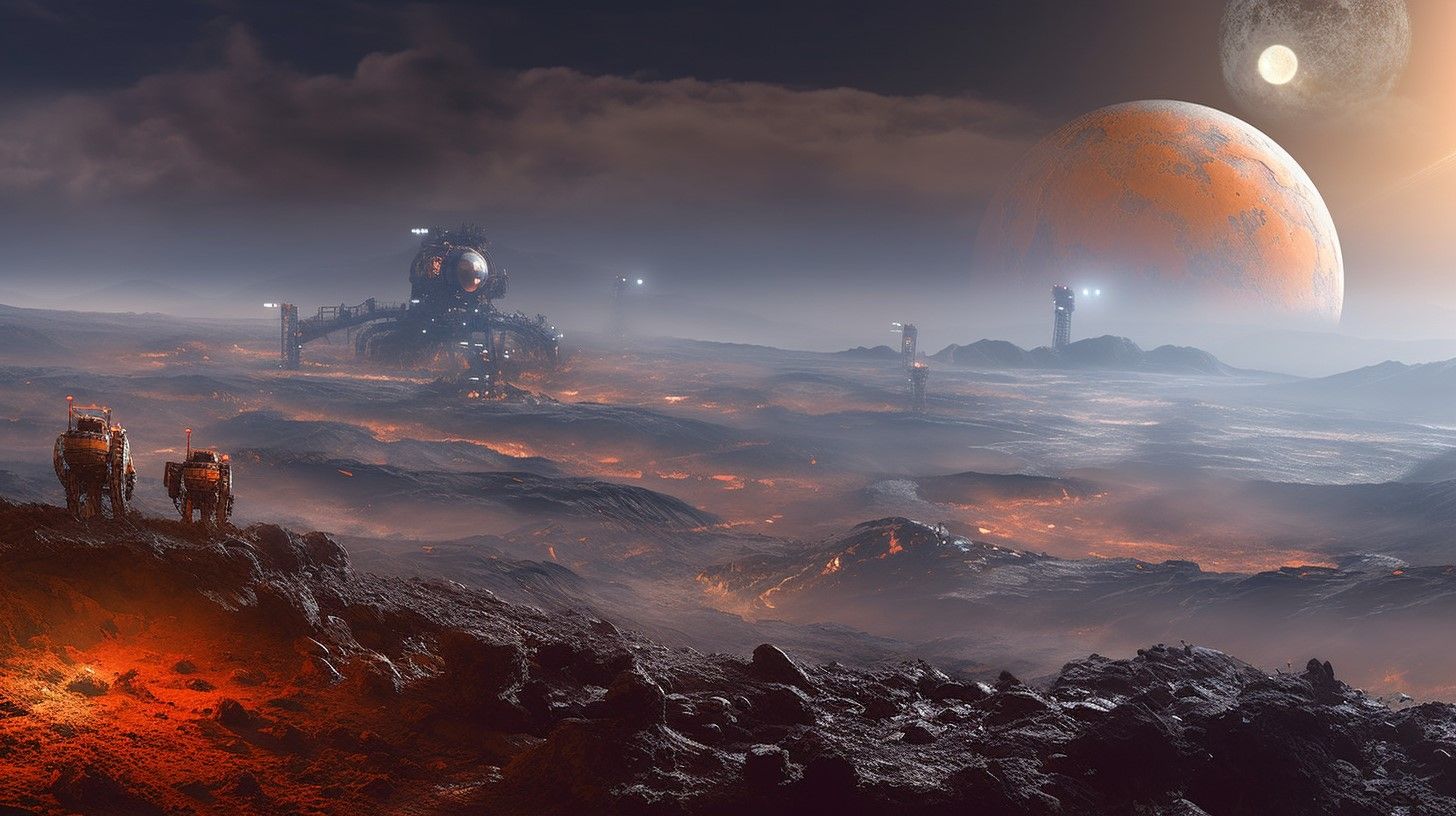
Looking further out, if we want to colonize Mars and reach across the stars, we are going to need AI to fly spaceships and terraform planets to make them habitable for humans.
So, all in all, I expect that:
AI, very much like globalization, will increase humanity’s overall wealth, accelerate progress, and help us conquer space while disrupting (sometimes painfully) many aspects of our society along the way.
Short term
In the short term, the key question is: Which jobs will this AI workforce perform? I think the first step toward answering this question is to ask, “How much can we trust AI workers to make decisions and in which contexts?” I believe reliability is the factor that will determine where AI can be used.
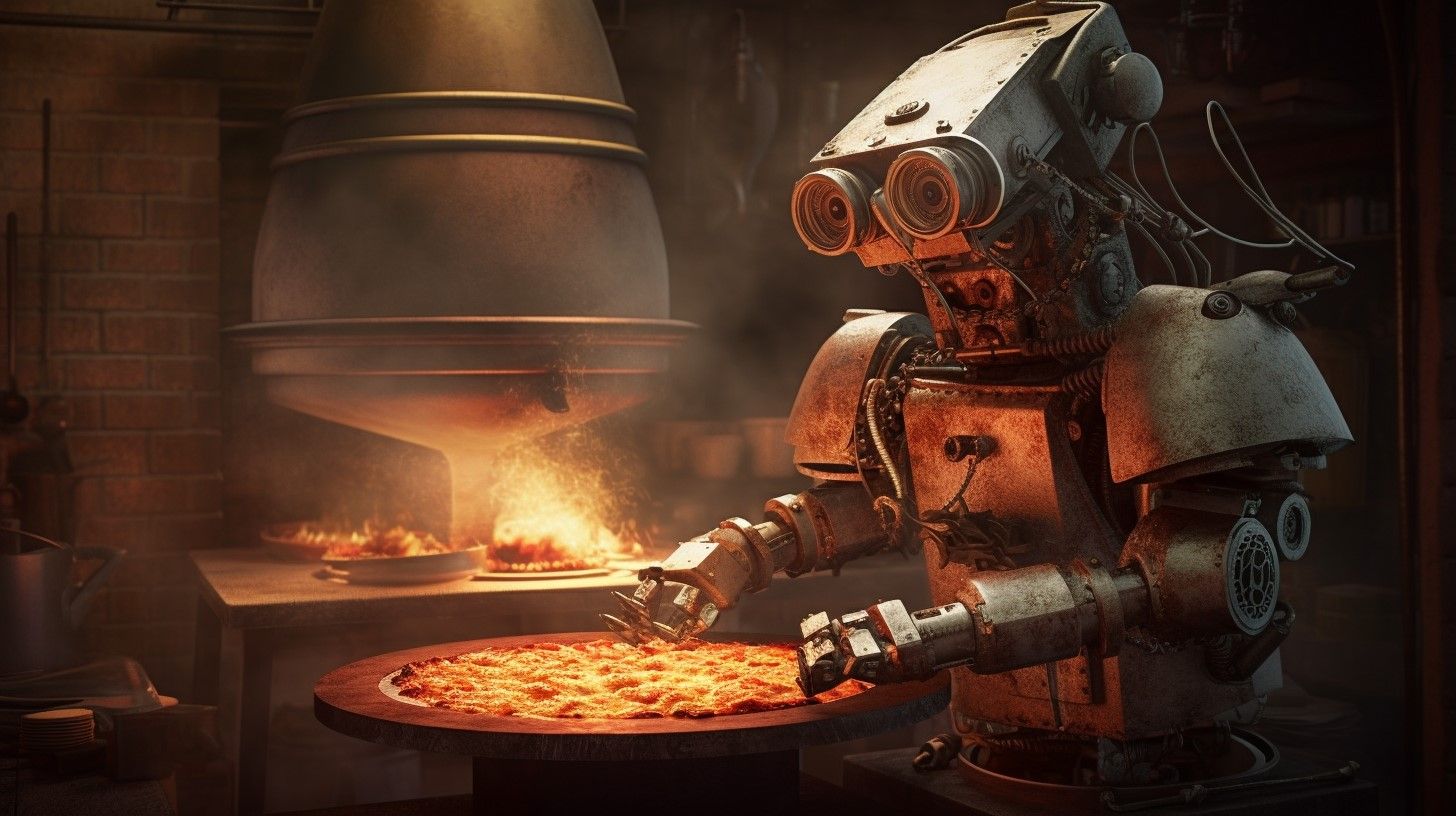
To illustrate why reliability and trust is the key to where AI will be used, let’s take the simple example of pizza ordering: In theory, we could have AI workers answer the phone and place the orders. This is achievable today, with current AI capabilities, but before pulling the trigger, companies will need to know how many mistakes the AI is going to make and if their consumers will be willing to tolerate that error rate.
I don’t have an answer for how many times people will be willing to receive the wrong pizza or not receive it at all because it was sent elsewhere due to AI mistakes before they switch to another pizza company. However, my guess is that consumers’ error tolerance will be very limited, given how reliable pizza orders are and the low cost of switching to a different pizza joint.
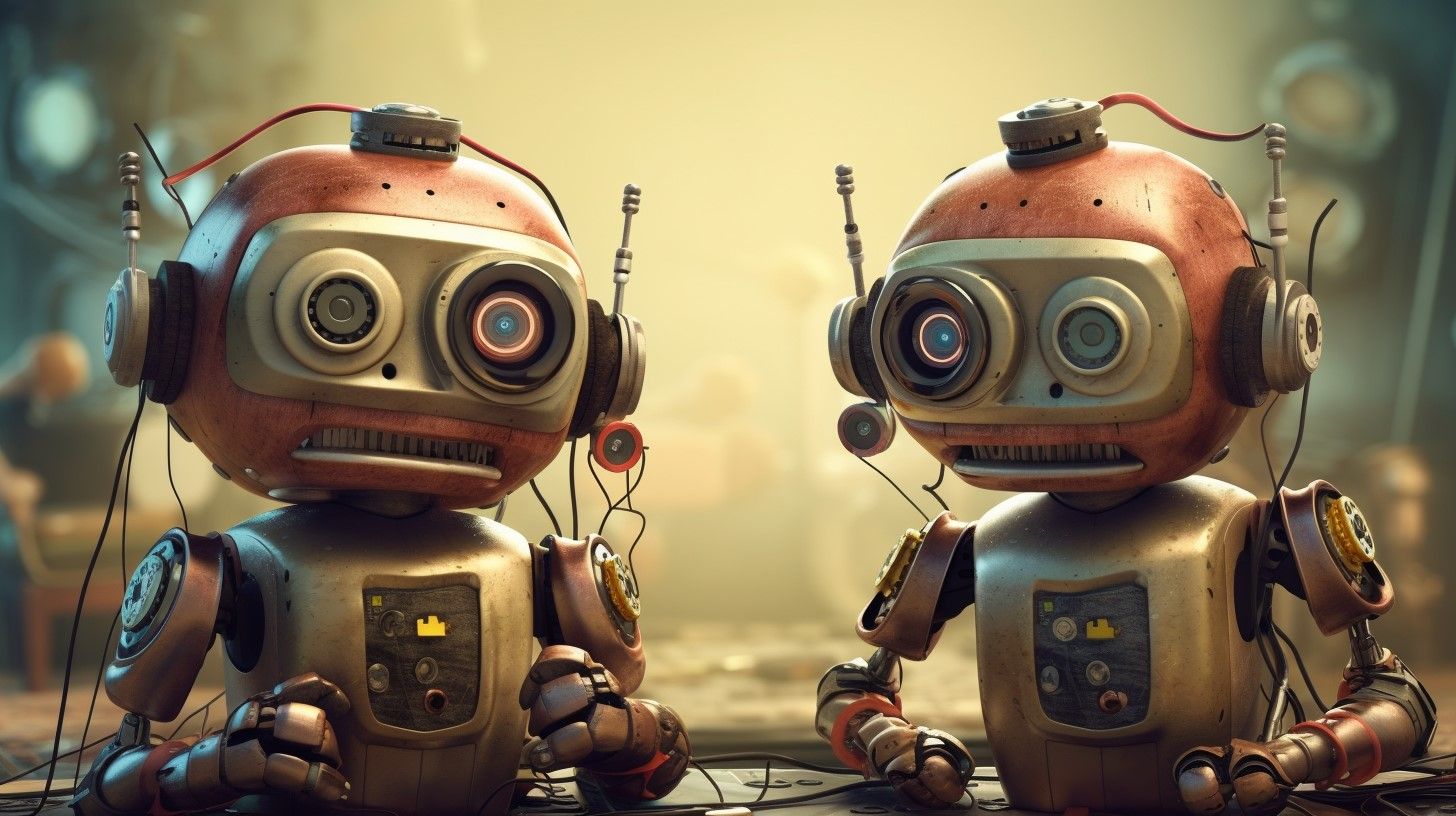
The same dilemma, trying to find a balance between reducing cost using AI and losing consumers due to AI mistakes, applies to most industries to various degrees: How many errors can AI bank agents make, such as suspending a card or opening the wrong account, before consumers switch to other banks? How about mobile operators and tech support? Are you willing to stay with a company if an AI decides to suspend your line, the next AI decides to not reinstate it, and you have to escalate for weeks?
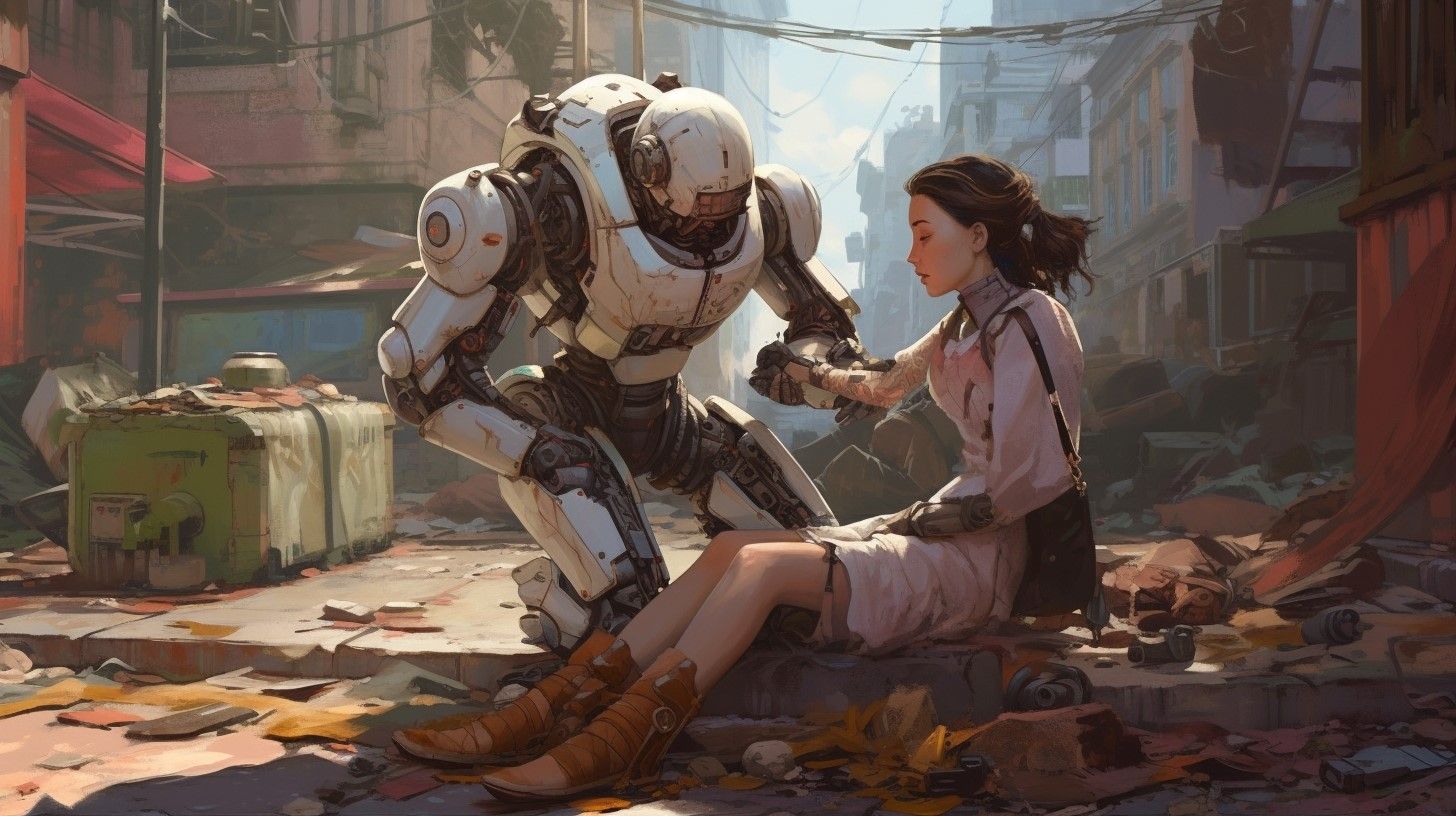
For some industries, such as health care, the legal and human cost of making mistakes is so high that AI reliability will have to be close to flawless. Those industries rely on multiple people to make decisions, so having them be automated seems, at least in the short term, very unlikely. For example, despite the life-saving benefits of instant health care provided by AI nurses, particularly in the face of disasters or pandemics, very few humans would be willing to be treated if the risk of medical errors is not very close to zero.
The error rates of AI workers will dictate how many jobs they can fully automate - until that rate is low enough, they will only be able to assist humans and will need to be supervised.
Autonomous AI time horizon
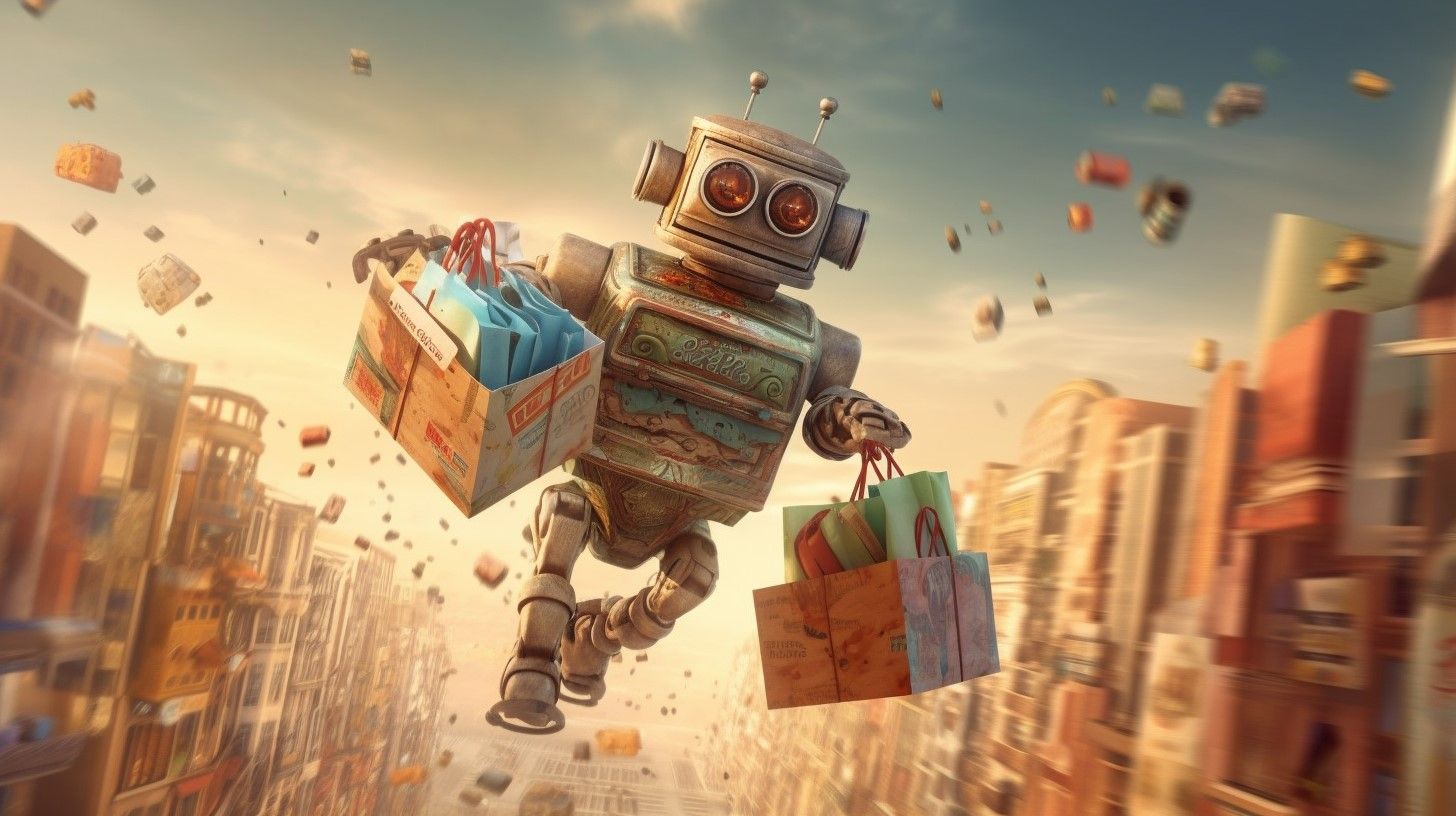
Based on how long the previous generation of AI took to develop, I believe that having an autonomous AI workforce will take at least a decade or more. Currently, GenAI seems to be squarely subject to the 20/80 Pareto principle:
We are experiencing 80% of the GenAI benefits by having done 20% of the work. Getting GenAI to be reliable will require completing the remaining 80%.
I think that, in that respect, GenAI is very similar to self-driving cars.
AI has been able to drive cars autonomously somewhat reliably for over a decade now (getting 80% of the results for 20% of the effort) but it is not until recently that AI has been able to reach a level of safety that is acceptable for general use.
AI workforce strengths
Let’s now dive deeper into why using the AI workforce is so appealing and will ultimately be adopted. Overall, AI workforce benefits can mostly be divided into four buckets:
GenAI white collar capabilities exceed what most humans can do.
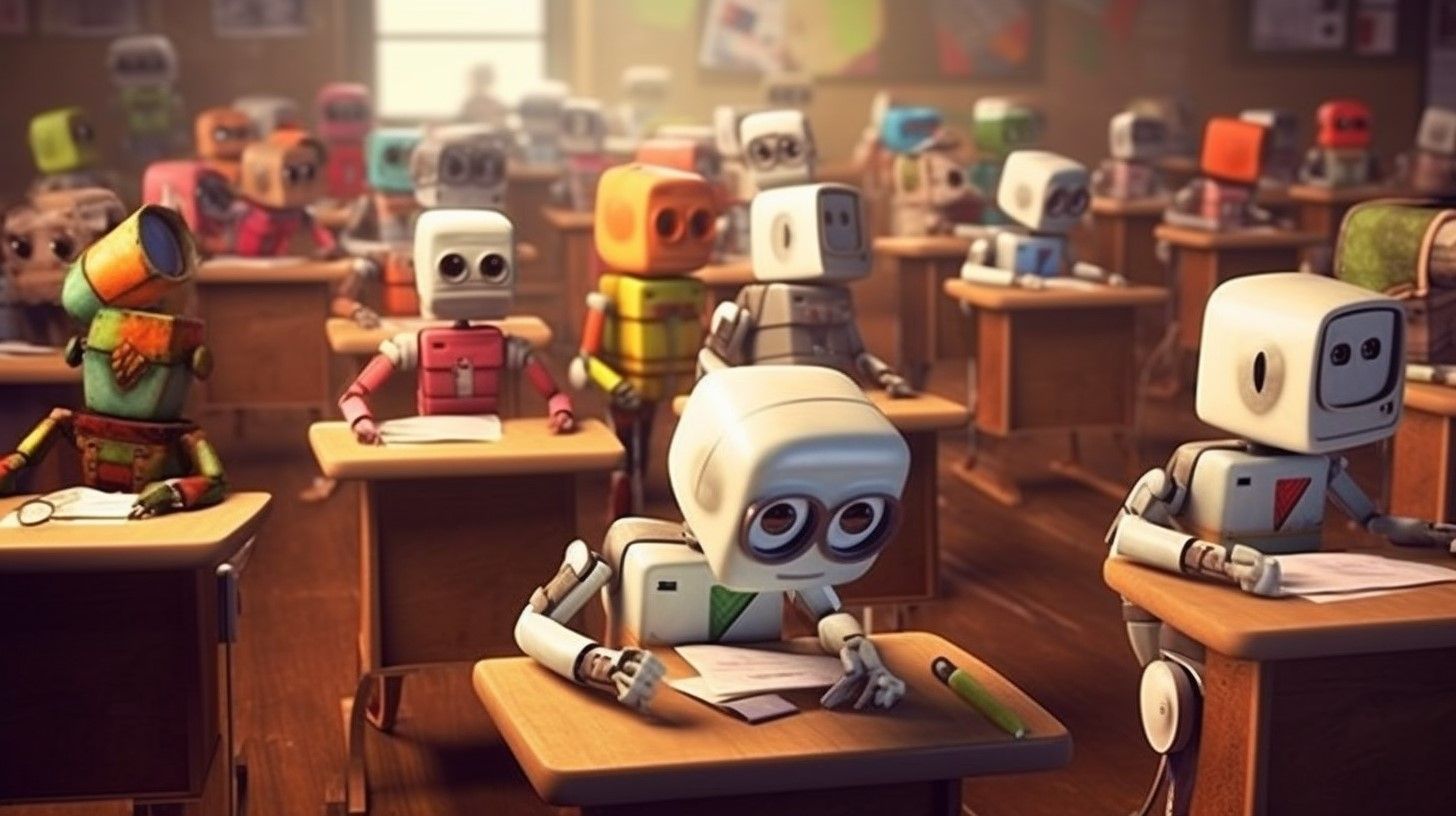
The GenAI models are able to create art and perform most knowledge-related tasks (e.g. writing, coding, answering questions) very well. For example, LLMs (large language models) are able to pass many human higher-education tests, including the SAT exam, the Bar exam, and theUSMLE (United States Medical Licensing Examination). That said, some of its claimed successes should be taken with a grain of salt due to flawed evaluations.
Artwise, it’s now the case that if you can imagine it, a text-to-image AI can create it – a case in point is the images contained in this blog post. However, you have little control over the details of the generated image, so it is not (yet) able to create an image that meets your exact specifications.
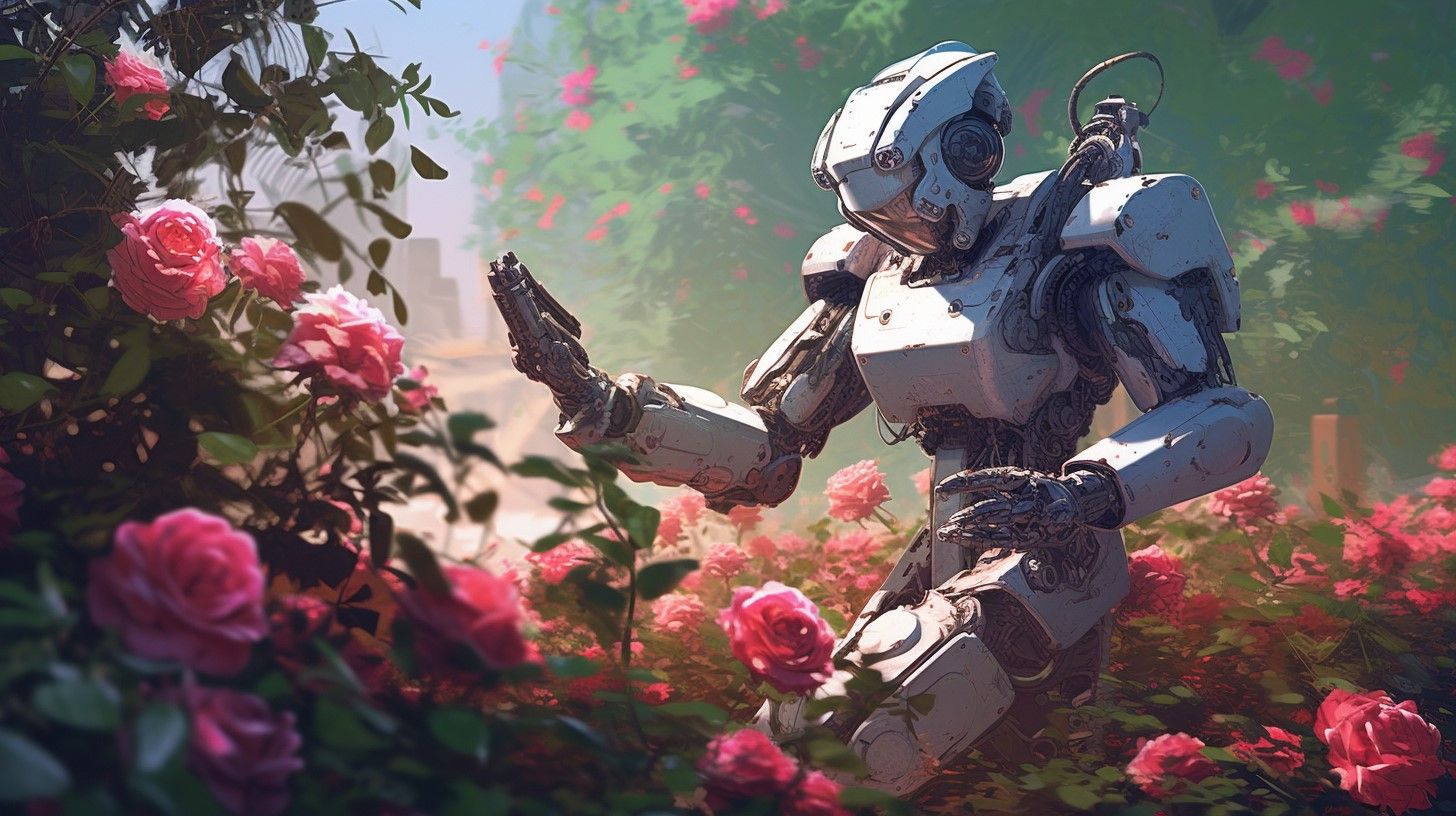
It is this ability to perform well in almost every field that makes GenAI more capable than humans and offers the hope that the AI workforce will be able to perform many jobs, including providing tech support, giving financial advice, being a shrink, teaching kids, being a paralegal, or acting as house clerks. Paired with advances in robotics, AI should also be able, one day, to take care of the most manually intensive work, such as construction, cleaning, and gardening.
GenAI is universally accessible.
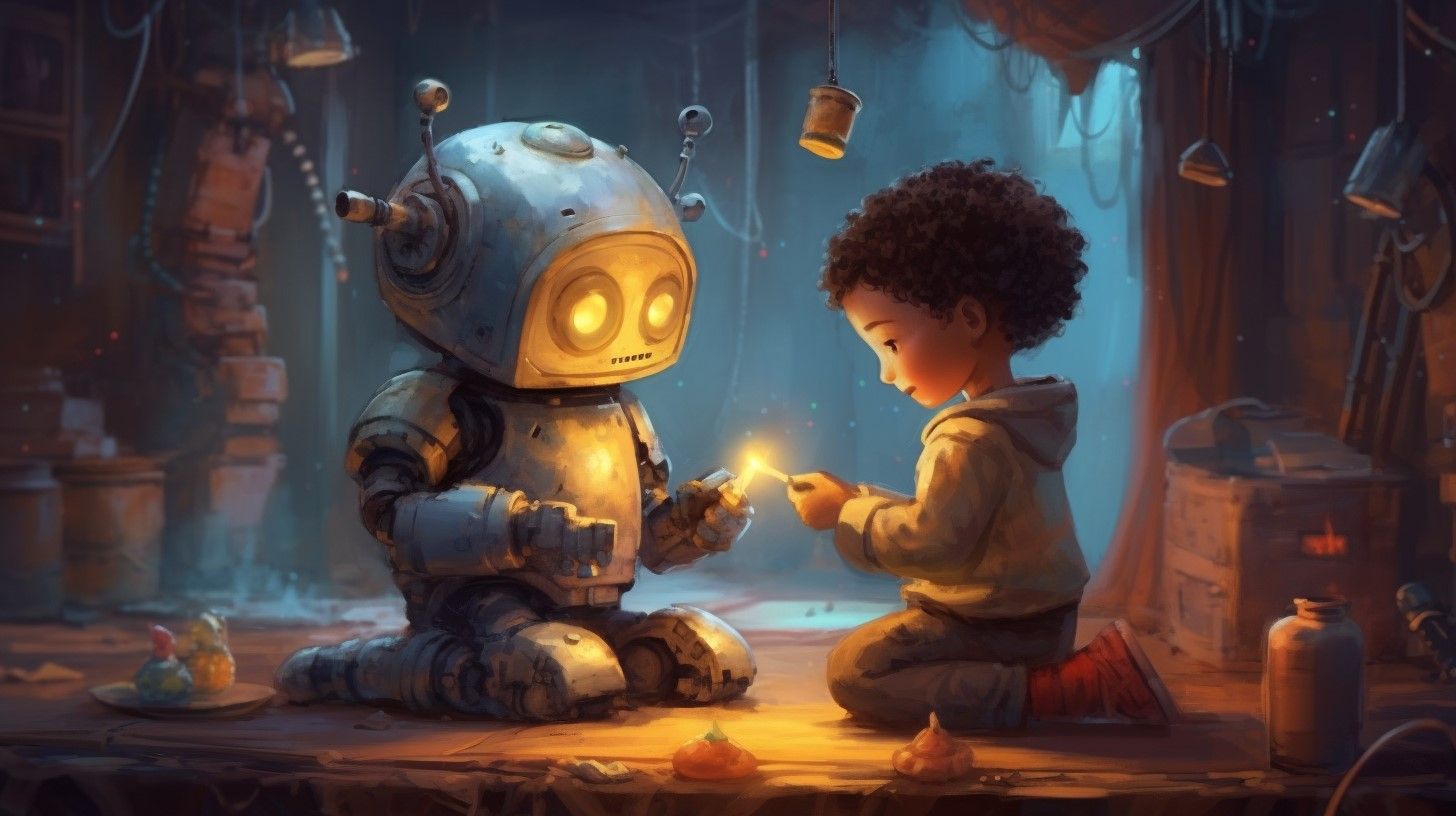
Simply type what you want and the AI will generate what you requested. Pair it with a voice recognition model and you can simply talk to it. It doesn’t get easier than that. The Nielsen Group calls this the first new UI paradigm in 60 years.
The fact that you can simply talk to a GenAI model as you would to another human makes it universally accessible.
Certainly there are techniques, such as prompt engineering to improve model performance, but those techniques are easy to learn and over time, models will become so good that such techniques will become useless - I suggest reading the famous bitter lesson blog post for a rationale on why handcrafted techniques always end up being replaced with more generic techniques.
AI is affordable.
Very large GenAI models that produce the best answers are quite hardware taxing – think tens of GPUs to get a response. However, this cost, a few cents, is drastically lower than any human wages for many tasks, making it cost-effective to use those models to assist human work as much as possible. This is very much like the Industrial Revolution, where using machines helped increase human throughput drastically.
AI is scalable.
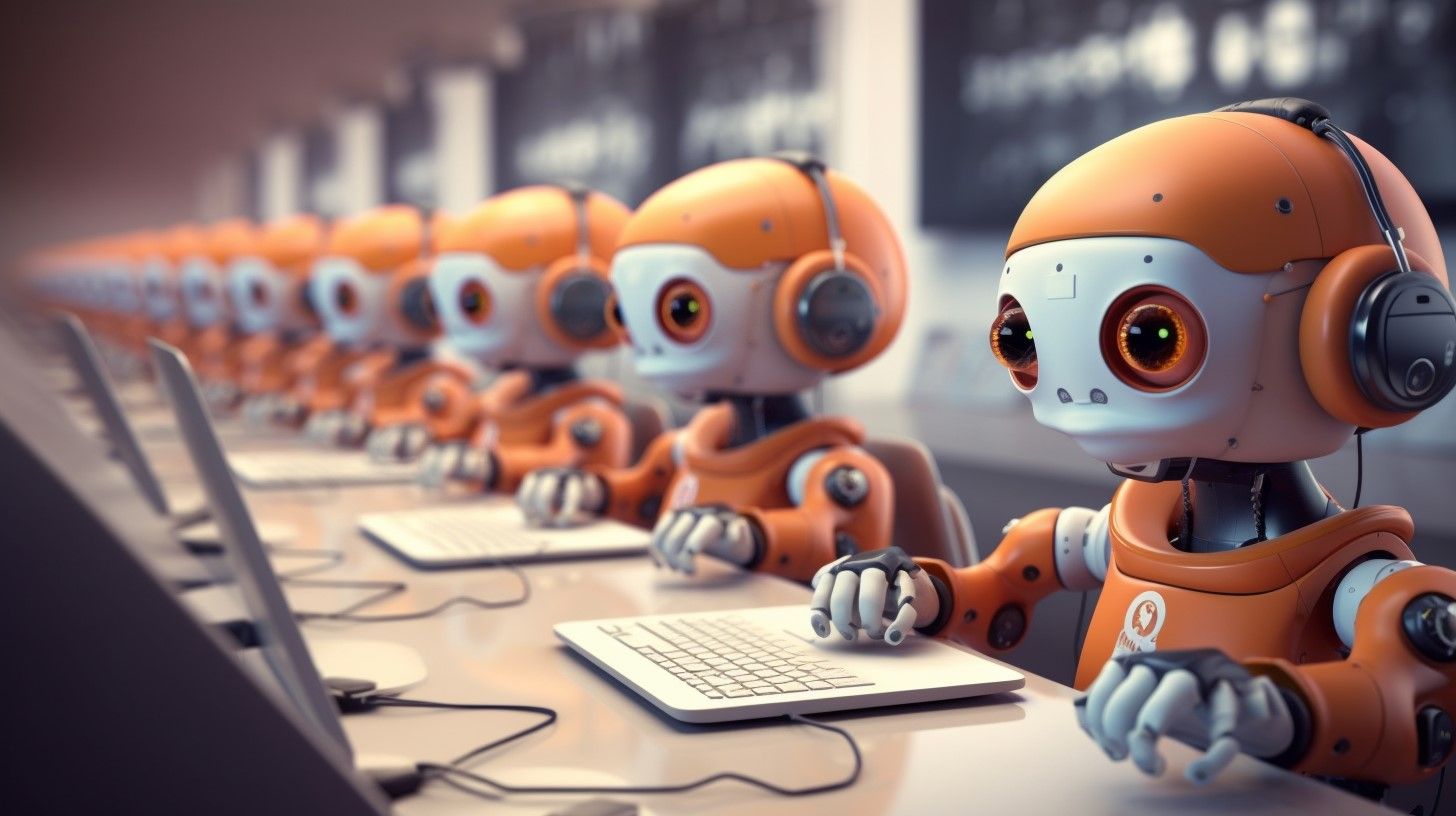
GenAI workers are readily available 24/7 to perform tasks at a moment’s notice on demand. Combine this elasticity with a low cost per task and you have unparalleled flexibility that scales with business needs. Today this scaling ability is somewhat limited by computer shortage but eventually, as more accelerators (GPU/TPU) come online, this shortage will disappear and the available pool of AI collaborators will become, for all intents and purposes, infinite.
AI workforce risks and challenges
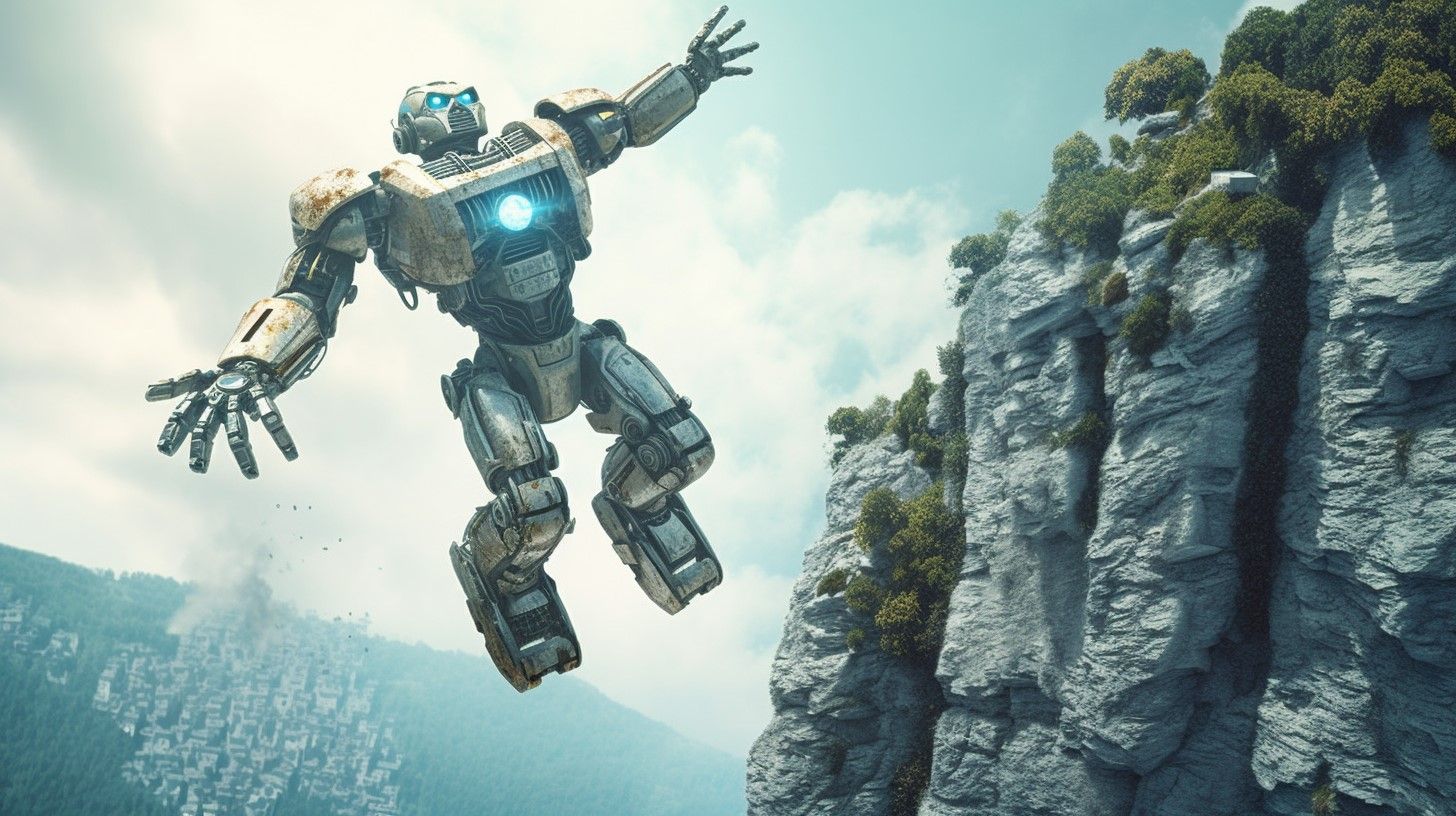
Despite large upsides, using an AI workforce comes with quite a few risks and challenges that might impede its short-term adoption. Those hurdles can be divided into roughly four buckets:
Reliability
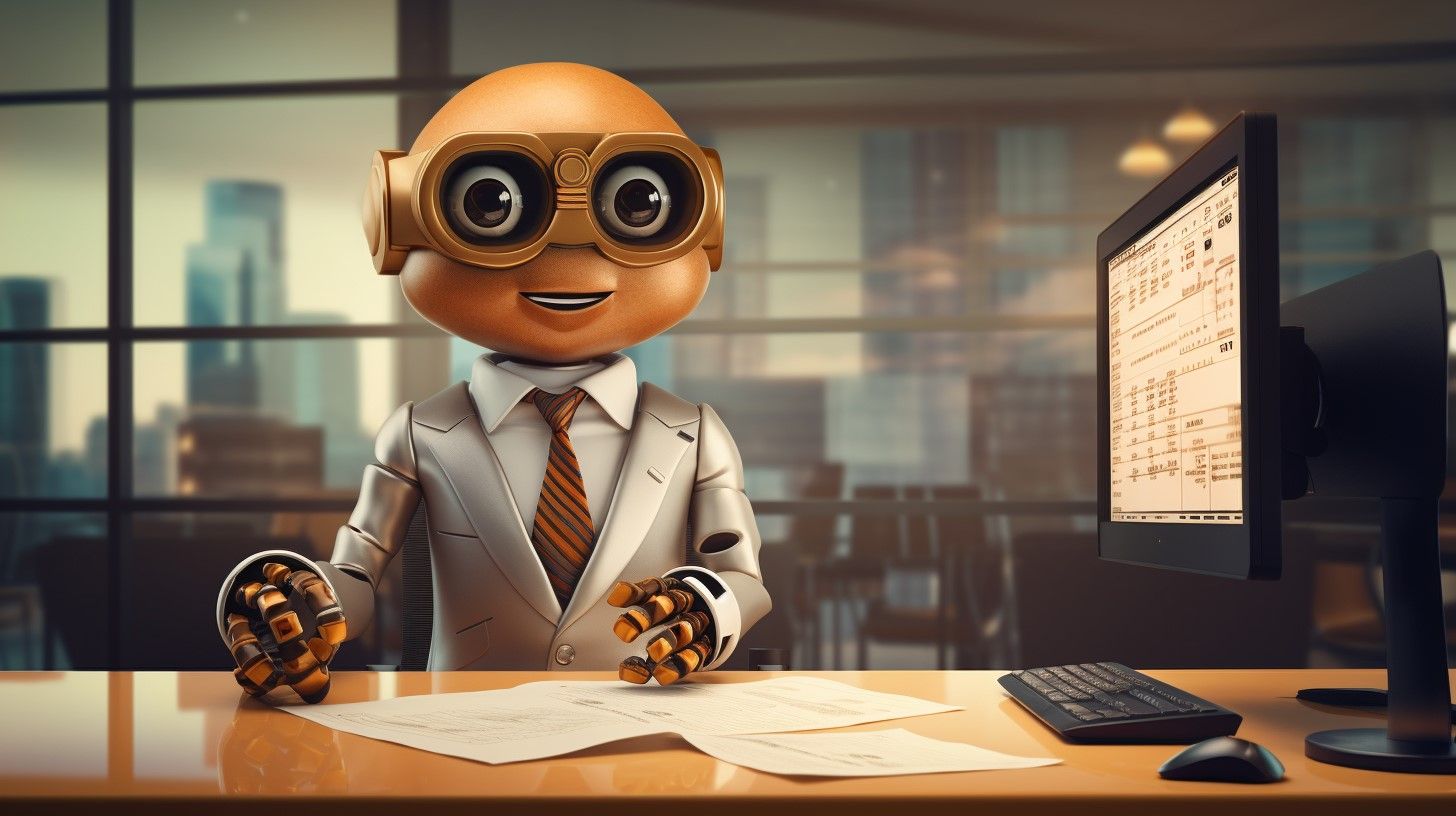
GenAI models are prone to “hallucination,” which is the technical term for making stuff up. In this respect, GenAI is not dissimilar to humans, who do this all the time when telling a joke, writing a fiction story, or lying on average at least twice a day.
The key difference is that (hopefully) most humans have a work ethic and understand when not to “screw up.” On the other hand, GenAI models have no concept of ethics or technical control mechanism (yet) to force them to make accurate statements. As a result, models make up facts and say them very confidently, which leads to content pieces that can be deceiving due to their strong wording. For instance, CNET had to correct more than half of the news written by GenAI and decided to pause using this technology.
While the need to have reliable consumer support and news articles is obvious, there are many other white collar jobs where accuracy is just as vital. For instance, consider marketing: Having an AI send a promotional newsletter with an unreasonable discount or a press statement full of inaccurate claims will have massive financial repercussions and potential legal ramifications – especially if the company is publicly traded.
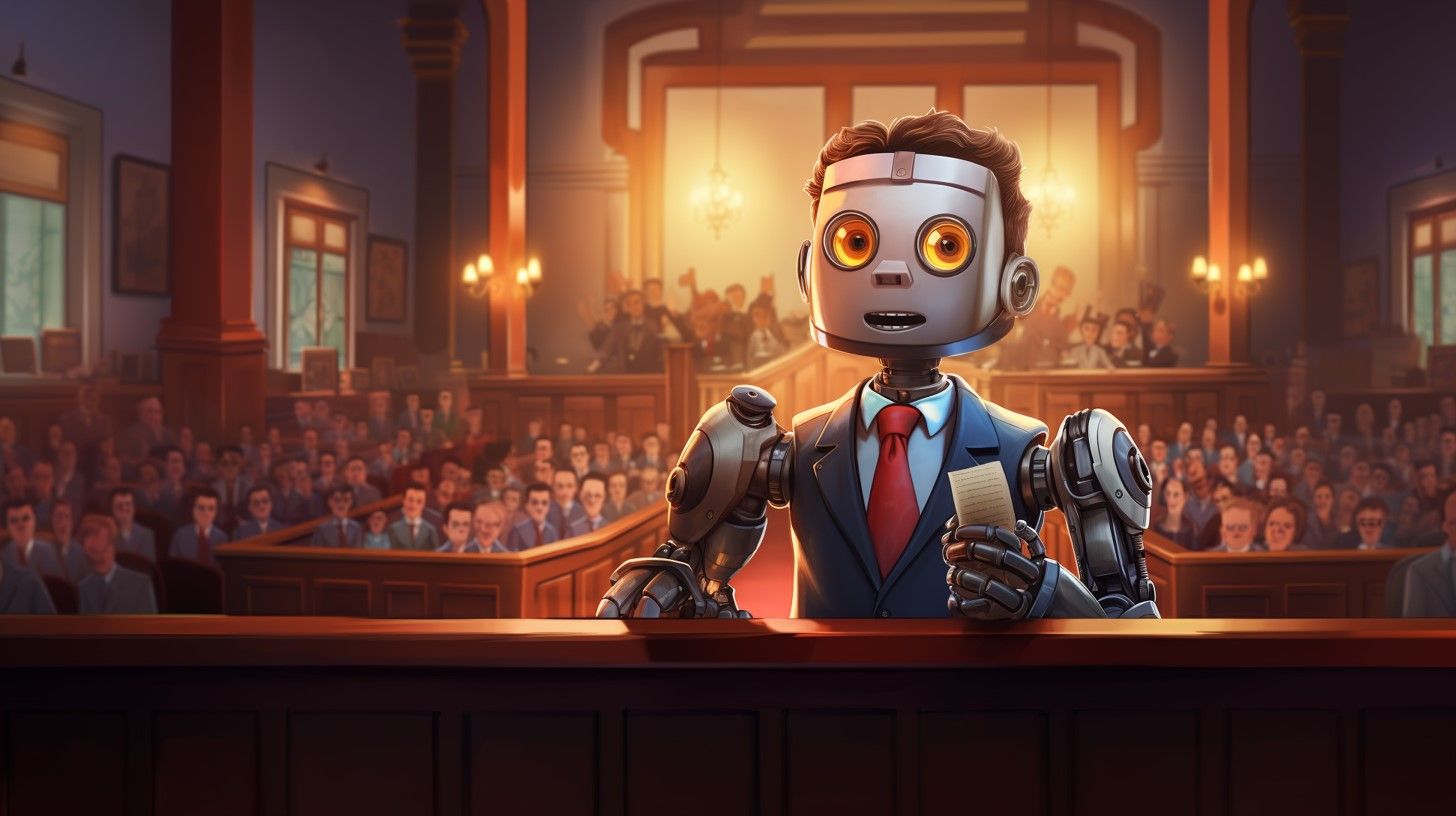
For many industries, such as defense, legal services, wealth management, transportation, and health, a single mistake is very costly, both in human and financial terms. For those industries, the required level of reliability will be even higher. This is why I don’t expect fully AI-automated workflows until AI workers incredibly reliable, as the cost of mistakes is either too high (as it is for health care, legal, and defense applications) and it’s too easy for users to switch to other providers (news, delivery services). I do expect a few companies to try anyway, but doing so will hurt their business significantly, as their consumers will be displaced, their competitors will use those mistakes against them, and they will be potentially sued. [Edit] Turns out this is already happening and went as badly as expected: Lawyers used [ChatGPT to find previous court cases relevant to a filing] (https://www.nytimes.com/2023/05/27/nyregion/avianca-airline-lawsuit-chatgpt.html). The AI made cases up, they didn’t check, and they got fined for it. After this warning shot, it is likely that the next mistakes will be met with harsher consequences, as pleading “I didn’t know” won’t work this time.
Overall, there are very few industries, if any, with lock-in strong enough that consumers will tolerate repeated mistakes and continue to remain clients. The collapse of Silicon Valley Bank clearly demonstrates this, with consumers fleeing incredibly quickly due to lack of confidence despite the cost of switching banks.
Effectiveness
While GenAI has demonstrated its effectiveness in speeding up image-related workflow, such as 3D assets texturing, its effectiveness at increasing productivity while assisting humans on other tasks remains to be demonstrated. For example, early research shows that developers using AI copilot are making more mistakes and only low-skilled consumer support workers benefited from AI assistance.
Stereotypes & Biases
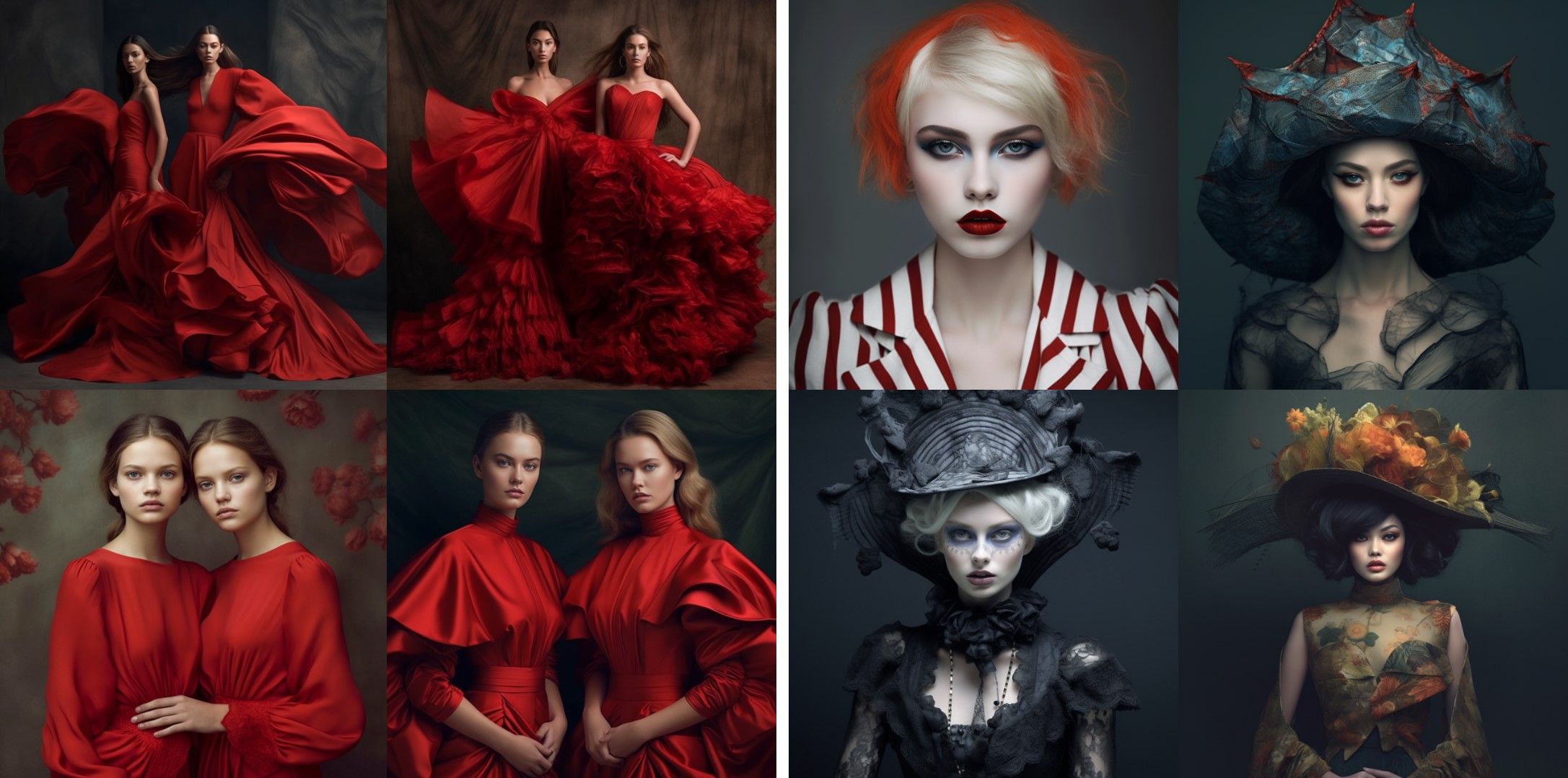
GenAI models need vast amounts of human-generated content for training, including trillions of tokens (words) and hundreds of millions of images. To meet those data needs, models are trained on a vast amount of Internet content, which causes them to inadvertently learn and internalize many stereotypes and biases that are subsequently reproduced in the content they generate. Such behavior is detrimental to society, as GenAI-generated content ends up perpetuating and even amplifying biases and stereotypes.
For brands, the risk of utilizing GenAI models that can potentially generate stereotyped and biased images and text is significant, as it will result in content that fails to be representative of their consumer base.
For instance, I generated the images above by requesting “fashion models in red dresses” and “fashion model.” As you can see, the images that were generated all have the same apparent gender, skin color, and body type. This lack of diversity is clearly undesirable, both for brands that cater to a broad consumer base, and for those targeting niche audiences that are distinct from the one portrayed in the generated content.
Creating generative AI that is inclusive and bias-free is going to be very difficult, as curating content at an extreme scale is not an easy task. Potential solutions include using AI to refine the training data, fine-tuning curated data to limit biases, and incorporating safety mechanisms into the model (e.g. negative prompt, safety classifier).
Originality
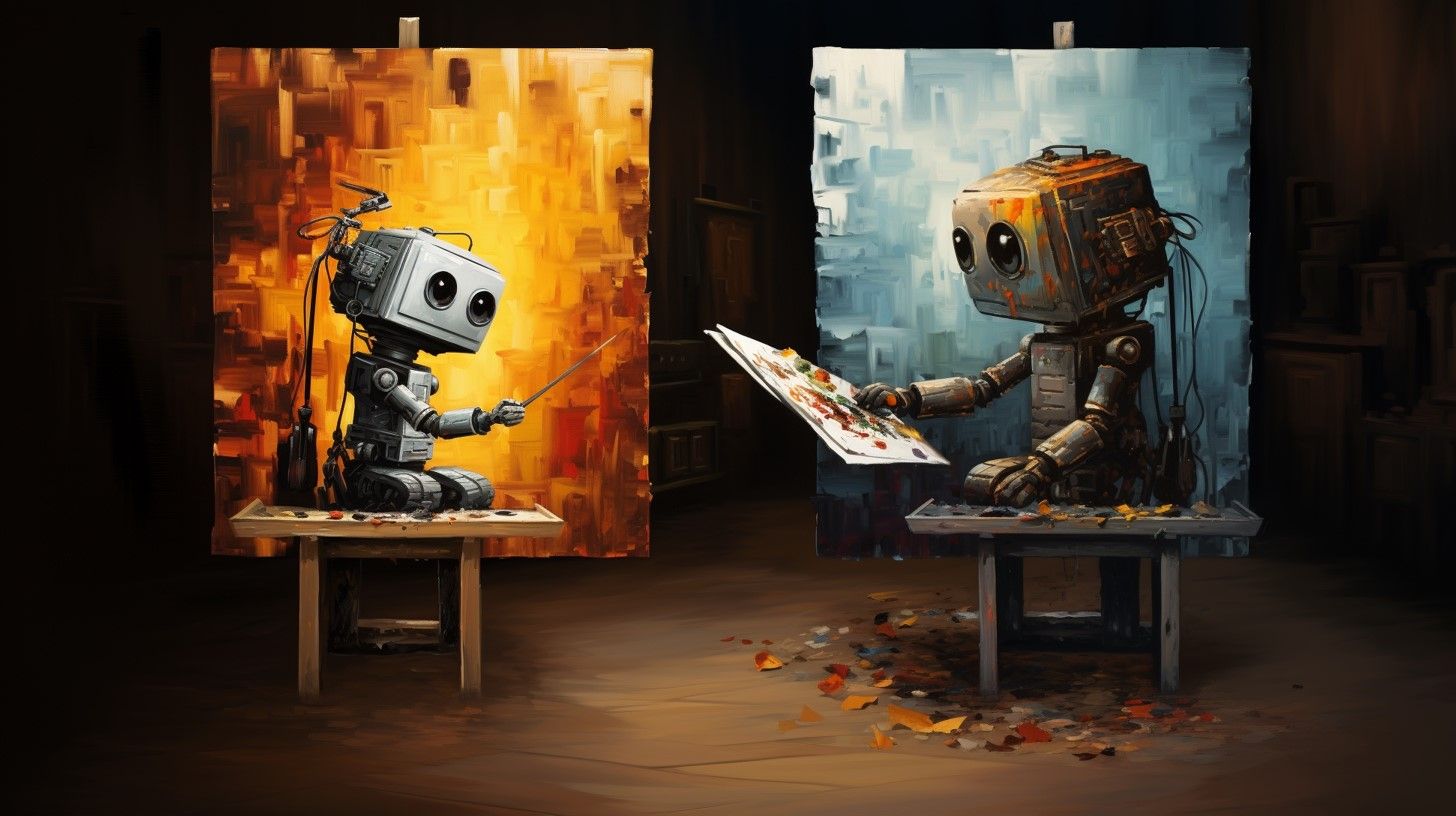
The key risks of training on human content are plagiarism and lack of originality. While for brands, using plagiarized content is a clear legal risk, lack of originality is equally deadly. If brand-generated content looks the same as everyone else’s content that was generated using the same models, brands risk becoming dull and losing their identity. Figuring out how to deal with this issue will probably require, at least, adjusting copyright laws, improving the technology with better safeguards, teaching AI to cite its sources, and new forms of content licensing.
More generally, it is unclear how original a GenAI model can be - they are certainly capable of generating novel content that is suitable for many applications, such as game textures, blog post illustrations, and email replies, but can GenAI generate truly innovative content with a unique and consistent style? This remains an open question.
Takeaways
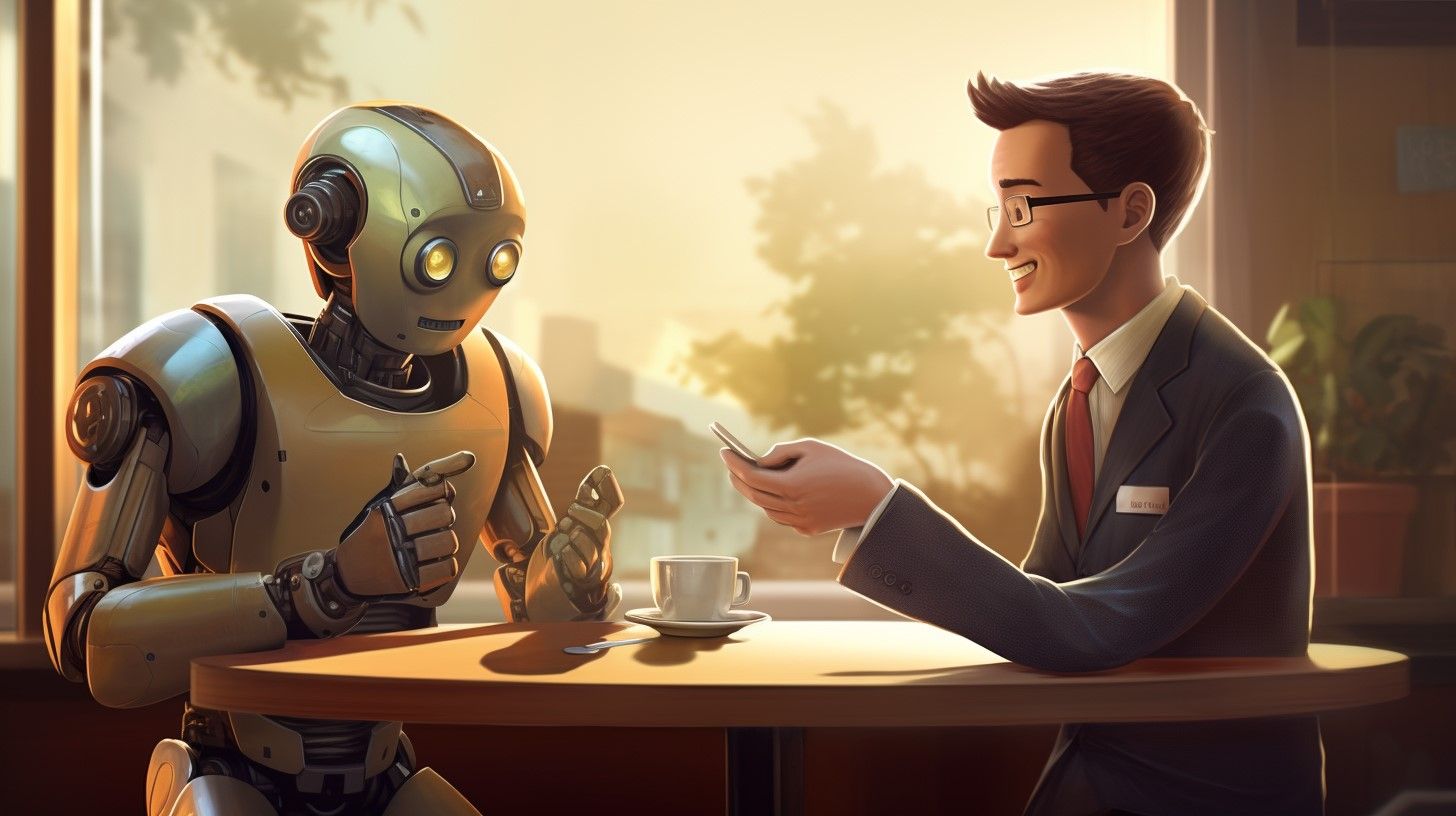
The AI workforce entering the market is full of promise, given its expertise, scalability, and affordability. In the long term, humanity is in dire need of this workforce taking an active part in society to face the challenges of declining human population, increasing living standards/needs, and the will to reach across the stars. Short-term reliability is going to be the key determining factor of how fast the AI workforce will be adopted. Until reliability is significantly improved, it is likely that AI collaborators will be mostly used to speed up human workflows, very much as mechanical machines, born out of the Industrial Revolution, do.
Thank you for reading this post till the end! If you found this article useful, please take a moment to share it with people who might benefit. To be notified when my next post is online, follow me on Twitter, Facebook, or LinkedIn. You can also get the full posts directly in your inbox by subscribing to the mailing list or via RSS.
A bientôt 👋